Entropy-based imagery segmentation for breast histology using the Stochastic Fractal Search
Breast cancer is one of the leading causes of death for women around the world. Its early diagnosis can significantly enhance the survival rate of the patient. Image processing techniques are used to help to diagnose this disease. The analysis of breast tissue samples on histological images is a challenging task where computer vision techniques can contribute. In this paper, the Stochastic Fractal Search (SFS) algorithm is applied to the image thresholding problem on breast histology imagery using as objective function three different entropies. The SFS is a new Evolutionary Algorithm (EA) which emulates the growth mechanism of fractals. SFS has been successfully applied to other applications, but its performance on image thresholding is unknown. The implementation of SFS is conducted using as objective function three of the most representative entropies being Kapur, Minimum Cross Entropy, and Tsallis. To provide a comparison point, two EAs commonly used for image thresholding are selected; the Artificial Bee Colony (ABC) and the Differential Evolution (DE). In this context, the resulting nine combinations are evaluated concerning the quality of the segmented images. Since the nine evaluated methods share either EA or entropy, the nonparametric test of Kruskal–Wallis is conducted to analyze the similarity of the results among methods. Results indicate that the combination of SFS and Minimum Cross Entropy yields the best results for the segmentation of histological imagery.
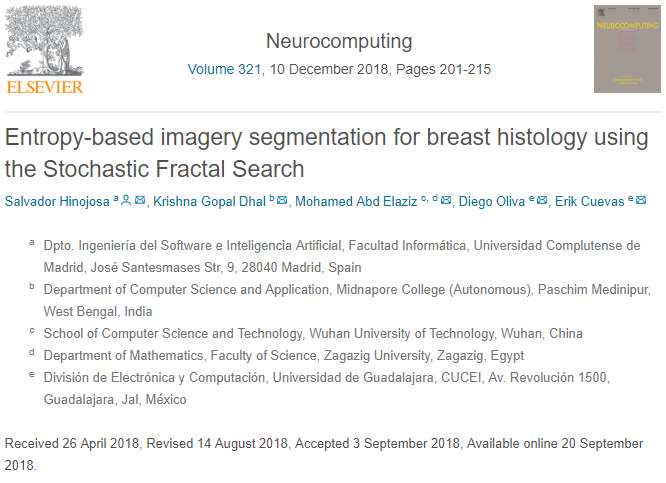
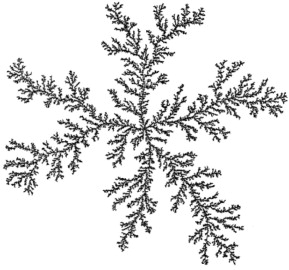
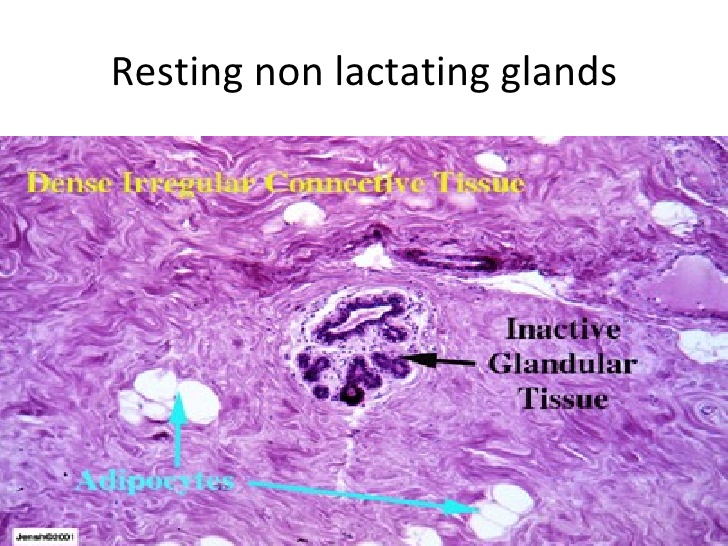